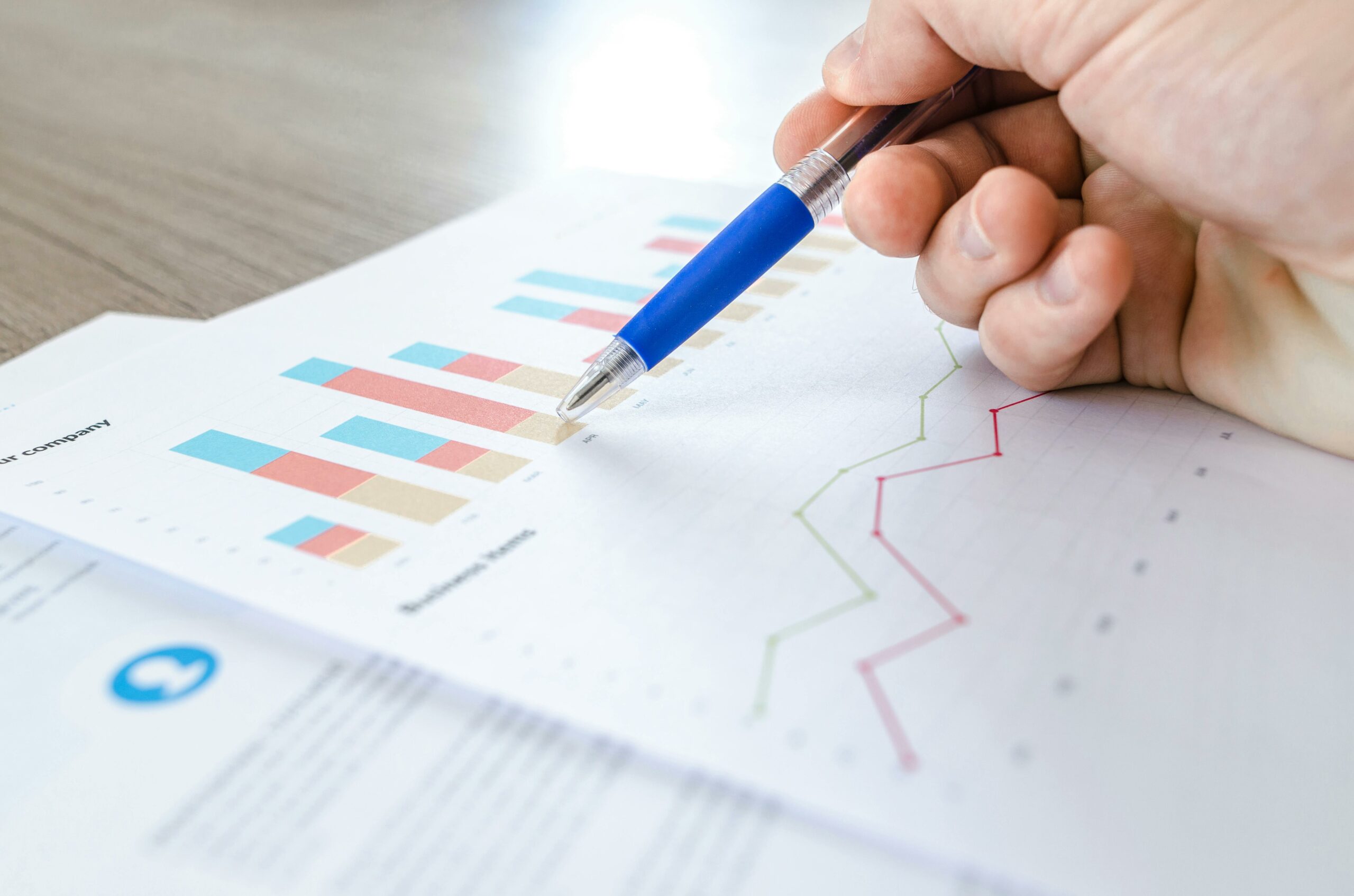
In any study, data is the backbone. When you’ve collected high-quality data, it’s easier to run analyses and present convincing conclusions. But, aside from measures to reduce missing or incomplete data, you also have to take steps to counter bias in your data, more specifically, information bias.
What is Information Bias? Who’s Messing With My Data?
Information bias is one of the most critical, yet underestimated types of bias in health research. According to Kesmodel (2018), this type of bias “occurs when any information used in a study is either measured or recorded inaccurately.” Simply put, information bias means that the information or data used by the research is incorrect (and so, obviously, the inferences the researcher draws from such data are also likely to be flawed).
You might encounter information bias when the data collected on exposure, outcome, and potential confounders is inaccurately measured. This can happen if someone unintentionally or intentionally misreports something that can’t be objectively verified, like frequency of intrusive thoughts.
Recording errors in self-administered questionnaires, interviews, or medical records can also contribute to this bias. For example, if drug dosage is recorded in milligrams instead of micrograms, this can significantly distort your findings.
Additionally, unstandardized data collection methods by different individuals can lead to misinterpretation of information. For example, one person may consider 1 alcoholic drink per day to be “moderate drinking” whereas another may label this “heavy drinking”. In a multicenter study, different institutes may collect and record data in different formats, resulting in inconsistencies. Clinicians may also unintentionally or intentionally assign outcomes based on prior knowledge of exposure, or fail to properly register exposure based on prior knowledge of an outcome, leading to biased results.
Lastly, during analysis, categorizing continuous data can introduce information bias. Let’s take the example of researchers analyzing the relationship between maternal age and neonatal morbidity. If the population is categorized as below 25, 25-29, 30-34, 34-39, and 40+, this categorization may lead to bias, because the outcomes for teen mothers (a known risk group) are conflated with those of women 20-24 years old.
Types of Information Bias: How Does My Data Get Messed Up?
As you design and conduct your study, you’ll encounter potential information bias in different forms:
Recall Bias: My Memory is Not What It Was
According to Tenny et al. (2023), recall bias is “the increased likelihood that those with the outcome will recall and report exposures compared to those without the outcome.” For instance, if you’re asking participants with and without gestational diabetes to report on how frequently they had influenza during adolescence, it’s possible that the participants with gestational diabetes have already been thinking deeply about what could have caused their condition and hence are more likely to remember influenza episodes. Here, recall bias could affect the data and lead you to conclude that frequent episodes of influenza during adolescence are a risk factor for gestational diabetes.
Observer Bias: What I Think Affects What I See
To overcome the limitations of self-reported data by participants, many researchers turn to using trained observers to collect data. However, this method isn’t foolproof because the data are still subject to observer bias, wherein outcome assessments are systematically influenced by the observers’ conscious or unconscious beliefs. More often than not, the observers hold some hopes or expectations in favor of the intervention, which leads them to overestimate the effect of the intervention.
Of course, one would ideally “blind” observers to which participants are receiving the intervention. But in some fields, such as physical therapy or medical device testing, this kind of blinding isn’t always feasible.
Performance Bias: The Trial Has Effects I Didn’t Anticipate
When you’re conducting a trial, you obviously try to make sure that there are no obvious differences between the intervention and control groups with regard to any factors that could affect the results (e.g., age distribution, comorbidities, etc.). But sometimes, the act of conducting a trial itself leads to differences between the two groups other than the intervention that’s being studied. To understand this better, let’s take a study on a weight loss intervention that involves regular meetings with a dietitian. Because the intervention group members are frequently coming to the study center to meet the dietitian, they encounter each other more frequently, become collegial, and start supporting each other in losing weight. Therefore, another factor, group support, is also aiding the weight loss efforts of the intervention group, not just the meetings with the dietitian.
Regression to the Mean: Everyone Becomes Average Sooner or Later
Suppose a group of researchers are testing a new small-molecule analgesic. They administer the drug to a sample of patients with severe pain. When they collect data post-intervention, pain scores have decreased drastically. However, some of this improvement could be due to regression to the mean: a statistical phenomenon wherein a second measurement of any variable for a specific group will be closer than the first measurement of that variable to the population mean. In other words, some of the decrease in pain scores could be due to random factors or just good luck. It’s important therefore for the researchers to “separate” the actual effects of the treatment from regression to the mean.
One of the most obvious ways of countering regression to the mean is to use a randomized controlled trial. When participants are randomly assigned to the intervention or control groups, you can more confidently attribute any improvement or decline in the intervention group to the actual intervention you’re studying. If using a control group isn’t feasible, you can take multiple baseline measurements when selecting your sample. You can then select participants on the basis of average measurements, not just a single score.
Leave a Reply