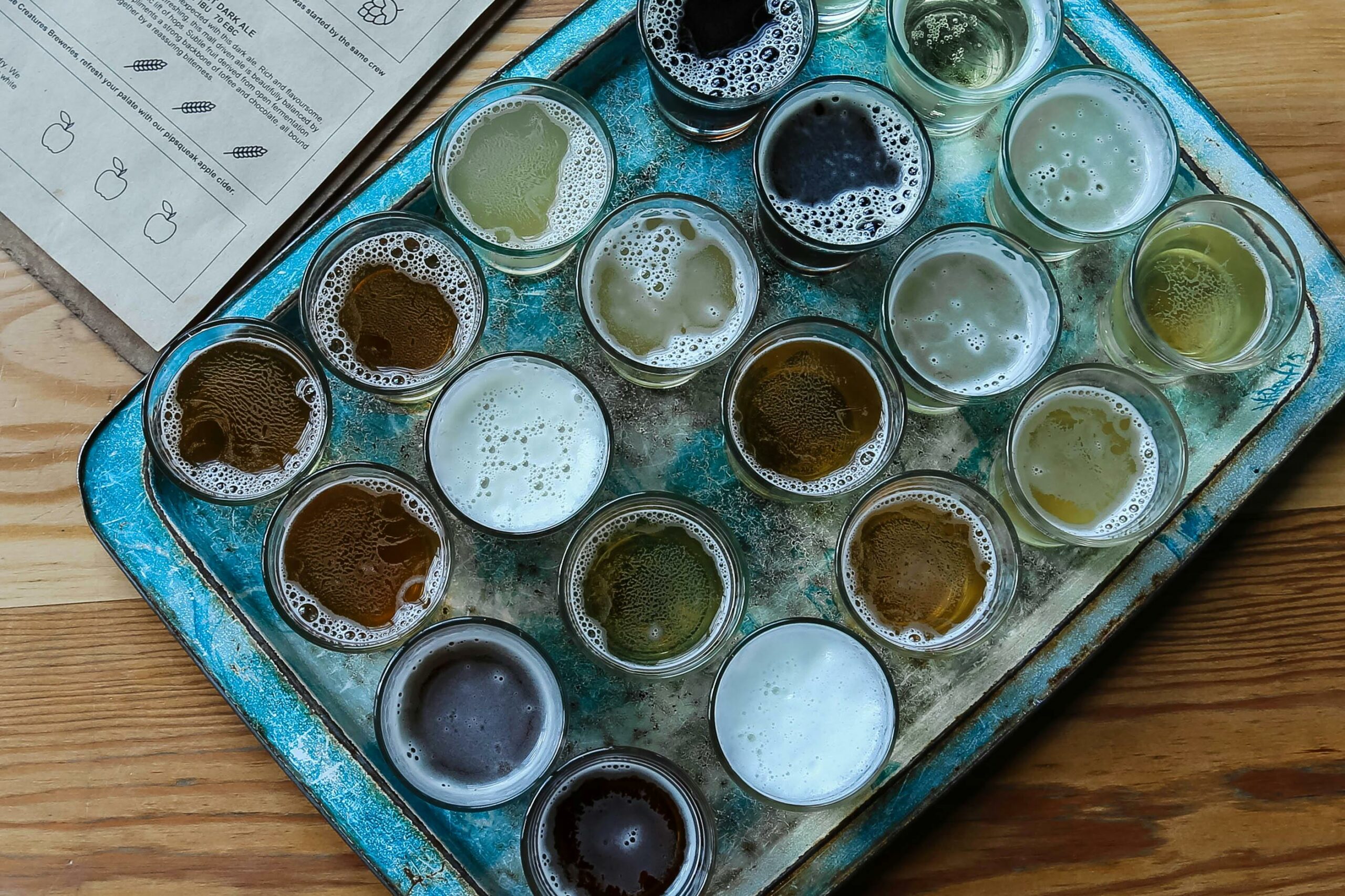
Getting your Trinity Audio player ready...
|
Have you ever wondered why some interventions seem like magic bullets in research papers, only to fizzle out in real-world applications? Selection bias might be the culprit.
What is Selection Bias?
Selection bias refers to a systematic error that creeps into research when the study sample isn’t representative of the larger population you’re interested in. Imagine studying the effectiveness of a new exercise program, but only recruiting highly motivated gym rats. Their results wouldn’t reflect the experience of the average person, leading to skewed conclusions.
Types of Selection Bias
Here’s how selection bias can infiltrate academic research:
- Self-selection: Participants might choose to join a study because they already have strong opinions or experiences relevant to the topic. For example, a survey on healthy eating habits might attract more health-conscious individuals, overestimating the overall population’s dietary choices.
- Sampling bias: The way researchers recruit participants can introduce bias. Relying on online surveys might exclude people without internet access, skewing demographics. Convenience samples, drawn from readily available populations like university students, might not generalize to the broader public.
- Measurement bias: The way data is collected can also be problematic. Surveys with leading questions or poorly defined answer choices can nudge participants towards specific responses. If researchers rely solely on self-reported data, participants might unintentionally misremember or embellish their experiences.
The consequences of selection bias are far-reaching. Published research with skewed results can mislead policymakers, healthcare professionals, and the public. Ineffective interventions might be adopted, wasting resources and potentially harming individuals.
So, how can researchers combat selection bias?
- Randomized controlled trials (RCTs): The gold standard for minimizing selection bias, RCTs randomly assign participants to treatment and control groups, ensuring baseline equivalence. While RCTs are powerful, they’re not always feasible or ethical in all research areas.
- Propensity score matching: This statistical technique attempts to create comparable groups in observational studies by matching participants on relevant characteristics.
- Transparency and acknowledgement: Researchers can be upfront about the limitations of their sampling methods and potential for selection bias. This allows readers to interpret the results with a healthy dose of skepticism.
Selection bias is a complex issue, but by being aware of its pitfalls and employing robust methodologies, researchers can produce more generalizable and reliable knowledge. Ultimately, this fosters a more trustworthy foundation for evidence-based decision making across all academic disciplines.
Remember: When encountering academic research, consider the potential for selection bias. Ask yourself: who participated in the study? How were they recruited? A critical eye helps ensure that research findings accurately reflect the real world, not just a select segment of it.
Leave a Reply